Workshop on "AI and Data Science for Earth System Sciences"
This workshop featured a series of presentations that explored the integration of AI and data science with Earth system sciences, highlighting innovative approaches and methodologies. We were eager to dive into these topics and engage in meaningful discussions on how AI and data science were advancing our understanding of Earth systems.
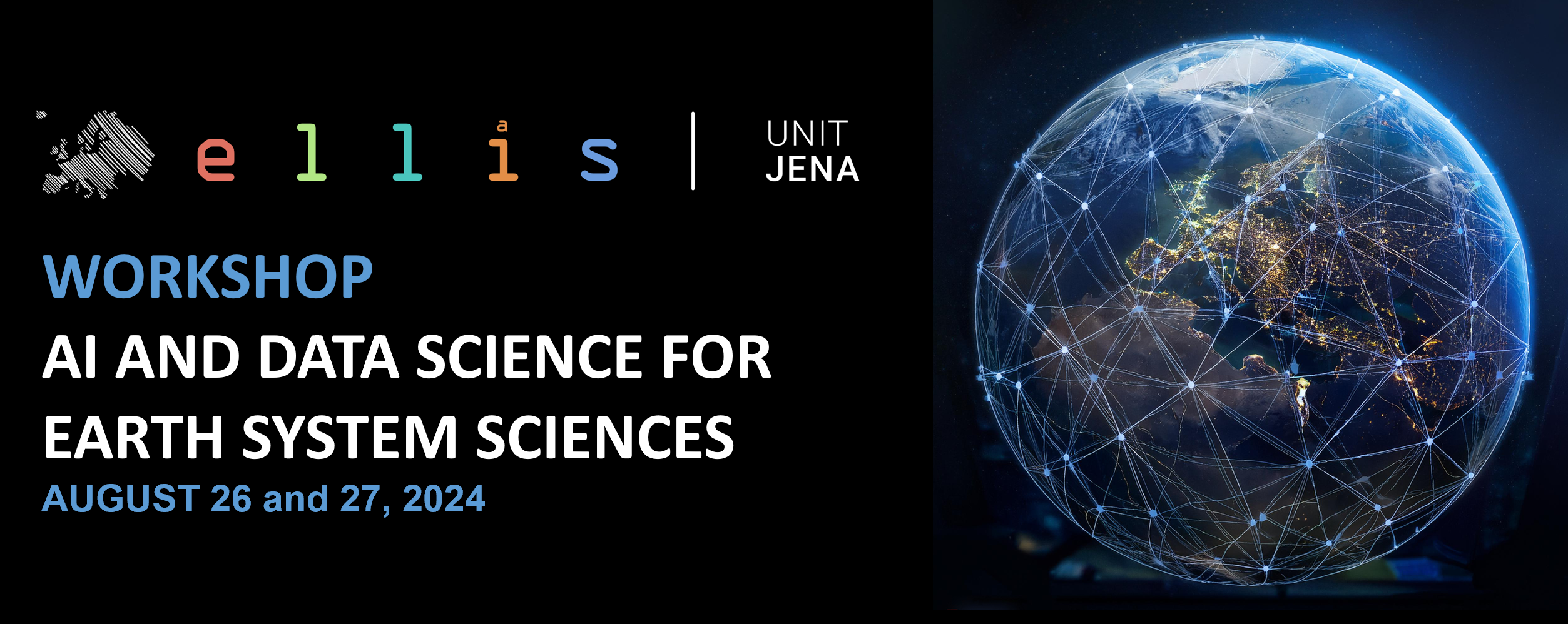
Location: Mälzerstraße 5, 07745 Jena at the IT-Paradies, 6th floor
AGENDA | DAY 1 - 26th AUGUST, 2024
REGISTRATION, LUNCH, NETWORKING, AND SEATING
12:00 - 13:00
WELCOME AND LOGISTICS
13:00 - 13:05
SESSION ONE 13:05 - 15:10
Causal Reduction of complex systems’ simulations
Anticipating how a real-world complex system behaves when external or internal properties change is key to our ability to understand it and inform policy decision. In absence of experimental data, gathering such causal knowledge is typically achieved by performing scientific simulations, which arguably entail causal knowledge. However, as scientific models become more intricate, deciphering the causes behind phenomena in high-dimensional spaces of interconnected variables becomes increasingly challenging. We investigate principled ways to extract interpretable causal knowledge from a low-level “microscopic” scientific simulation by mapping it to a causal model comprising a reduced set of interpretable high-level “macroscopic” variables. A key learning signal to find such a causal reduction it the approximate causal consistency between the low-level causal model, and the high-level causal model we want to uncover. As a first approach, we have introduced Targeted Causal Reduction (TCR), a method for condensing complex intervenable models into a concise set of causal factors that explain a specific target phenomenon. We proposed an information theoretic objective to learn TCR from interventional data of simulations, establish identifiability for continuous variables under shift interventions and present a practical algorithm for learning linear TCRs. Its ability to generate interpretable high-level explanations from complex models is demonstrated on toy and mechanical systems. Ongoing work now investigates non-linear TCR, the learning of causal reductions without specifying a target, and how these methods may help close the gap between scientific simulations and real-world systems.
13:05 - 13:30
Interpretable Machine Learning for Advancing Watershed Modeling and Understanding
Interpretable Machine Learning for Advancing Watershed Modeling and Understanding Interpretable machine learning has emerged as a powerful approach for gaining insights from data by elucidating the underlying mechanisms captured by data-driven models. In the context of watershed systems - complex entities that are critical for providing freshwater resources for energy production, agriculture, industry, and human consumption - this approach holds great promise. In this talk, we will explore how interpretable machine learning can improve our predictive understanding of the dominant processes governing the hydro-biogeochemical functions of watersheds in diverse environmental settings. By using these models, we can deepen our understanding of how these critical systems respond to varying environmental conditions, ultimately informing better management and conservation practices.
13:30 - 13:55
Harnessing Kolmogorov Arnold Networks to Explore Phenological Responses to Climate Change
Understanding phenology—the timing of biological events in relation to climate—is essential in a changing climate, as it affects carbon and water exchange between the biosphere and atmosphere, species interactions, and agricultural productivity. However, the specific responses of phenology to ongoing climate changes remain largely unknown, creating uncertainty in predicting ecosystem resilience and adaptability. This study addresses critical questions regarding the drivers of variability in vegetation greenness, focusing on the contributions of current weather conditions versus memory effects. We investigate the extent to which immediate meteorological influences and historical conditions contribute to variance in greenness, disentangling the vegetation-memory effect from meteorological memory. We place particular emphasis on the beginning and end of the growing season, as these periods are crucial for understanding seasonal dynamics. Additionally, we explore the implications of global warming and frost events on vegetation health, examining how uniform temperature increases and late-spring frost events, both individually and in combination, affect vegetation dynamics. To achieve these objectives, we train Kolmogorov Arnold Networks (KANs) to predict vegetation color indices from meteorological variables. A key advantage of KANs is their ability to separate the influence of different variables, providing an intuitive and systematic method for quantifying variable importance. Furthermore, we enhance interpretability and generalization by integrating symbolic formulations into the KAN framework, creating hybrid models. By comparing the outcomes of our experiments and analyses, we gain insights into the potential impacts of climate change on vegetation dynamics.
13:55 - 14:20
Hybrid-Modeling of Land-Atmosphere Fluxes Using Integrated Machine Learning in the ICON-ESM Modeling Framework
We are developing data-driven parametrizations for terrestrial carbon-water coupling using eddy-covariance flux measurements (FLUXNET) with a multi-task Feed-forward Neural Network (FNN) approach. Our hybrid model integrates data-driven and mechanistic elements, aiming to replace empirical parametrizations of photosynthesis (GPP) and transpiration (Etr) with FNN models trained on observations. Initially, we validate our approach by training parametrizations on JSBACH4 output, demonstrating that it effectively reconstructs original parametrizations like stomatal conductance (gs). We then replace JSBACH4’s parametrizations with emulator-based ones via a Python-FORTRAN bridge and assess their impact on the model output. Preliminary results show high R² values (0.91-0.99 for GPP and 0.92-0.99 for Etr) at half-hourly scales. We are now preparing the hybrid JSBACH4 model with parametrizations trained on FLUXNET observations, which will be used in coupled land-atmosphere simulations with ICON-ESM.
14:20 - 14:30
Causal-Inference in ecological data sources
While the positive effects of plant diversity are well-documented in ecological literature, understanding the underlying causal structure—specifically among soil, plant, and climate interactions—remains unclear. To address this, our work focuses on applying Causal Discovery methods to ecological data sources. As these methods often make strong assumptions about data distributions, their successful application involves not only interpreting results but also determining which methods are particularly reliable in this domain. Our work covers both areas by developing Causal Discovery benchmarks and analyzing ecological data provided by the Jena Experiment, one of the world's longest-running biodiversity experiments.
14:30 - 14:40
Q&A AND DISCUSSION SESSION 1
14:40 - 15:10
BREAK (COFFEE, TEA, SNACKS) AND POSTER SESSION
15:10 - 16:10
SESSION TWO 16:10 - 17:50
Mapping Glacier Basal Sliding Applying Machine Learning
During the RESOLVE project ("High-resolution imaging in subsurface geophysics: development of a multi-instrument platform for interdisciplinary research"), continuous surface displacement and seismic array observations were obtained on Glacier d’Argentière in the French Alps for 35 days in May 2018. This dataset is used to perform a detailed study of targeted processes within the highly dynamic cryospheric environment. In particular, the physical processes controlling glacial basal motion are poorly understood and remain challenging to observe directly. This is especially true in the Alpine region for temperate-based glaciers, where the ice rapidly responds to changing climatic conditions, making the processes strongly intermittent in time and heterogeneous in space. Spatially dense seismic and Global Positioning System (GPS) measurements are analysed using machine learning to gain insight into the processes controlling the glacial motions of Glacier d’Argentière. By using multiple bandpass-filtered copies of the continuous seismic waveforms, we compute energy-based features, develop a matched field beamforming catalog, and include meteorological observations. Features describing the data are analysed with a gradient boosting decision tree model to directly estimate the GPS displacements from the seismic noise. We posit that features of the seismic noise provide direct access to the dominant parameters that drive displacement on the highly variable and unsteady surface of the glacier. The machine learning model infers daily fluctuations and longer-term trends. The results show that on-ice displacement rates are strongly modulated by activity at the base of the glacier. The techniques presented provide a new approach to study glacial basal sliding and uncover its full complexity.
16:10 - 16:35
Identifying probabilistic weather regimes targeted to a local-scale impact variable
Weather regimes are recurrent and persistent large-scale atmospheric circulation patterns that modulate local impact variables such as extreme precipitation. However, existing methods for identifying weather regimes are not designed to capture the physical processes relevant to the impact variable in question while still representing the full atmospheric phase space. Here, we propose two novel machine learning methods, abbreviated as RMM-VAE and CMM-VAE, for identifying probabilistic weather regimes targeted to local-scale impact variables. Building on variational inference and a variational autoencoder architecture, the methods develop a statistically coherent model for combining non-linear probabilistic dimensionality reduction using a neural network with probabilistic clustering of the latent space using a mixture model, and a prediction task. The new methods are applied to identify circulation patterns over the Mediterranean region targeted to precipitation over Morocco and compared to state-of-the-art techniques. Our results show that the new methods identify clusters with higher predictive skill of the target variable, while also outperforming these two methods in reconstructing the atmospheric phase space. Our findings overall demonstrate the trade-offs involved in targeted clustering which can inform future method development in this area.
16:35 - 17:00
Exploring self-supervised learning for soil moisture dynamics
To address the limitations of traditional equation- and parameter- based models in capturing soil heterogeneity and the complexity of soil-water-atmosphere-plant interactions, machine learning models for soil water processes have been increasingly developed due to their advantage of data-driven process representation. However, current machine learning approaches for soil water processes rely on supervised learning, depending on specific labels to approximate moisture response at specific spatiotemporal points. This paradigm not only necessitates different models for predictions of different target space and time, but also fall short of essentially representing soil hydrodynamics. In this study, we explored the potential of self-supervised learning to extract generic representations of soil moisture dynamics, and examined the possibility of one single machine learning model capable of predicting soil water content across various depths and timeframes. Specifically, we 1) developed a transformer-based model to characterize spatiotemporal soil moisture transport, 2) evaluated the model’s spatiotemporal extrapolative predictions against supervised learning baselines, and 3) examined the interpretability of the latent representations in relation to diverse soil properties.
17:00 - 17:10
Predicting Vegetation Responses to Climatic Extremes Using Machine Learning
Forecasting the impact of climatic extreme events, such as droughts and heat waves, on vegetation is essential for effectively mitigating their effects on both human populations and biodiversity. However, understanding how these events influence vegetation at the landscape level remains limited, as their effects are highly heterogeneous depending on geographic locations, surrounding environments, and the history of extreme events in those regions. Recent advances in machine learning offer new opportunities to predict the impact of these extreme events. In this talk, I will present the Earthnet initiative, where we use machine learning to forecast the evolution of the Vegetation Index (VI) based on Sentinel-2 imagery, using previous Sentinel-2 sequences and meteorological information to guide the prediction. But, extreme events pose a significant challenge in machine learning due to their inherently imbalanced nature. The situation is further complicated by the lack of a clear method to quantify the impact of climatic extremes on vegetation at the global scale, which is crucial for evaluating machine learning model performance. I will discuss approaches to address this issue and present our preliminary results.
17:10 - 17:20
Q&A AND DISCUSSION SESSION 2
17:20 - 17:50
SUMMARY OF THE DAY
17:50 - 18:00
DINNER AND NETWORKING
18:00 - 21:00
AGENDA | DAY 2 - 27th AUGUST, 2024
DAY 2 OPENING REMARKS
8:30 - 8:35
SESSION THREE 8:35 - 10:05
An apparent multi-decadal global ocean cold anomaly in the early twentieth century temperature record
The observed temperature record, combining sea surface temperatures (SST) with near-surface air temperatures over land (LSAT), is crucial for understanding climate variability and change. However, early records of global mean surface temperature (GMST) are uncertain due to changes in measurement technology and practice, alongside incomplete spatial coverage. Here, using a statistical learning technique, we independently reconstruct GMST from SST and LSAT data. We find very high agreement between the two reconstructions, except during a large multi-decadal cold anomaly in the early twentieth century (1900-1930), which is on average about 0.26C colder in the ocean reconstruction compared to the land data. The ocean cold anomaly is unforced, but internal variability in climate models cannot explain the observed land-ocean discrepancy. Several lines of evidence based on attribution, time scale analysis, coastal grid cells and paleoclimate data support the argument that the observed global SST record is implausibly cold in the early twentieth century. While long-term warming since the mid-nineteenth century is not affected, correcting the ocean cold anomaly would result in a more modest early twentieth century warming trend, a lower estimate of decadal-scale variability inferred from the instrumental record, and better agreement between simulated and observed warming than existing datasets suggest.
8:35 - 9:00
The role of uncertainty quantification in building trust in machine learning models
Uncertainty Quantification (UQ) is crucial for improving the reliability and safety of machine learning (ML) models, particularly in safety-critical areas. In fields like remote sensing, ML models are extensively used to monitor environmental changes, and assess the impacts of climate change. However, without understanding how confident a model is in its predictions, these results can be misleading or even harmful. UQ addresses this challenge by ensuring that the model’s confidence is well-calibrated—meaning the confidence level accurately reflects the model's actual performance. UQ helps identify the sources of uncertainty, whether they arise from inherent noise in the data (aleatoric uncertainty) or from the model's limitations (epistemic uncertainty), and helps managing out-of-distribution (OOD) data, which are common in dynamic environments. This presentation will give a brief introduction to UQ and its importance in building trustworthy models. It will explore key methods for quantifying uncertainty in single-modality data, such as images, and in more complex systems that combine multiple types of data, such as Vision-Language Models (VLMs), that integrate visual and textual information to provide a more comprehensive understanding of the environment.
9:00 - 9:25
Leveraging Citizen Science and Machine Learning for Enhanced Plant Phenological Monitoring
Plant phenology, the study of timing in plant life cycle events such as budburst, flowering, fruiting, and senescence, is essential for understanding plant responses to environmental conditions. Despite increased interest in phenology, documenting these processes remains challenging due to their extensive spatial and temporal scales. Global phenological networks typically collect data at the individual level, but the decreasing number of phenological observers raises concerns about the future of these datasets. Concurrently, the widespread use of plant identification apps by the public has resulted in a vast collection of plant occurrence records and ccompanying images across diverse temporal and spatial scales. This study examines the feasibility of using opportunistically captured plant observations from a plant identification app to determine various phenological stages. Additionally, we investigate the potential for enhancing citizen science-based phenological monitoring by incorporating images gathered by these apps. We developed a machine learning-based workflow to automatically annotate thousands of images, categorizing them into specific phenological stages. Our methodology not only addresses the onset of flowering but also enables large-scale exploration of other stages, such as budburst and fruiting. We compare these opportunistic phenological records with systematically collected data from established phenological networks. Our approach not only streamlines the annotation process but also enhances the utility of citizen science data for phenological monitoring, offering a scalable solution for future research in plant phenology.
9:25 - 9:35
Q&A AND DISCUSSION SESSION 3
9:35 - 10:05
BREAK (COFFEE, TEA, SNACKS)
10:05 - 10:35
SESSION FOUR 10:35 - 12:25
Towards understanding weather drivers of large impacts with interpretable machine learning
High-impact weather events such as floods, weather-driven crop failure and forest mortality emerge from complex interacting weather drivers at different spatial and temporal scales. Machine learning models are able to learn such complex relationships, but their interpretation is challenging, and often data limitations contribute additional difficulties. In this talk I will present examples of (i) how knowledge about the analysed processes can help make the machine learning models more interpretable, (ii) how simulation data from process-based models can be used to test new analytical approaches, and (iii) how different cross-validation strategies can affect model performance estimates and their interpretation.
10:35 - 11:00
Identifying climate drivers of agricultural yield failure using interpretable machine learning
Agricultural yields are influenced by growing-season weather conditions in complex and nonlinear ways. For example, maize yield is adversely impacted by high temperatures, but this effect can be ameliorated by irrigation or high humidity, and the sensitivity of the plant to heat varies throughout its phenological cycle. Machine learning methods are able to learn such relationships, but extracting them from the trained model is difficult. Furthermore, complex models can rely on spurious correlations rather than the ‘true’ underlying mechanisms. Using simulated data from process-based crop models, we develop a method for extracting relevant climate drivers from high-resolution multivariate time-series data based on model performance in unseen climate conditions. This allows us to identify and inter-compare the relationships between growing-season weather and maize yield failure as embodied in current crop models and explore interactions between the identified drivers. Ultimately, we aim to present a robust method for the identification of compound climate drivers of crop yield failure that can be used on spatiotemporal observational data to improve our understanding of the impact of compound abiotic stresses on agricultural productivity.
11:00 - 11:10
Impact of Vegetation on soil moisture anomalies by atmospheric transport in South America
Recent decades have seen significant changes in soil moisture variability in South America due to ongoing greening and deforestation. However, research on the impact of vegetation on soil moisture anomalies (SMA) has largely been confined to local scales. Atmospheric transport can transfer these effects to downwind regions, altering soil moisture. In this study, we developed a spatially informed time series convolutional neural network model to link upwind leaf area index (LAI) with downwind SMA, guided by atmospheric moisture data using a mechanism attention approach. Our findings reveal that upwind vegetation explains soil moisture variability with an R² of 0.86. The Pampas region was particularly sensitive, receiving increased moisture from upwind LAI between 2001 and 2018. Additionally, vegetation in the southern Amazon had greater contribution to SMA in its downwind areas. We quantified that upwind vegetation mitigated adverse effects in 59% of drought events. Our results highlight the significant impact of vegetation changes and hopefully provide clear guidance for afforestation programs and agricultural water resource management beyond local and regional scales.
11:10 - 11:20
Bias in Neural Networks for Earth System Sciences
The black-box nature of most modern machine-learning methods makes it challenging to identify when models are influenced by unsuitable features or properties. Such biases can arise from various sources, inlcuding capture bias during data collection and spurious correlations (misleading associations). In areas where accurate predictions are critical, such as early warning systems, addressing these biases is crucial for developing robust and reliable machine-learning models.
11:20 - 11:30
Capstone talk
Machine learning in the exploration of biodiversity - climate interactions: plans and examples
Biodiversity decline and climate change are connected via intricate coupling mechanisms. On both ends, multiple sources provide a wealth of data, and machine learning approaches are required to optimally exploit these. The talk will present the ideas in this regard for a new cluster on the connection between biodiversity and climate developed between Jena and Leipzig. It will illustrate some previous work on classification in remote sensing, and on emulation in remote sensing and modelling.
11:30 - 11:55
Q&A AND DISCUSSION SESSION 4
11:55 - 12:25
CLOSING REMARKS
12:25 - 12:30
LUNCH AND NETWORKING
12:30 - 14:00
END OF THE WORKSHOP
14:00
Location: Mälzerstraße 5, 07745 Jena at the IT-Paradies, 6th floor
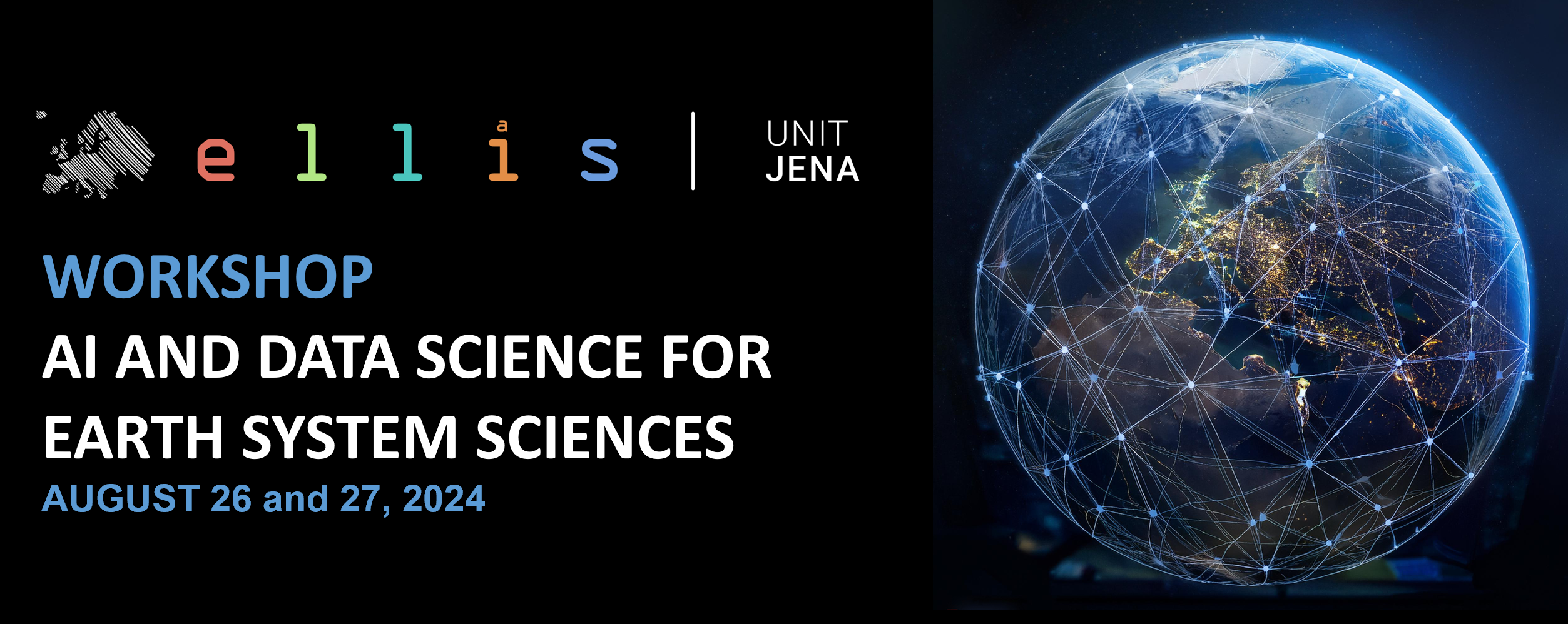