The research pursued by the ELLIS Unit Jena is at its core motivated by the desire to explore how environmental and climate sciences can profit from machine learning and AI advances to gain a better understanding of Earth dynamic systems. Highlights of this research agenda include fundamental and pioneering work on the evaluation of climate change impacts in the society and the economy. Beyond a purely scientific work, this research will enable the regulatory bodies and policy makers in their decisions and measurements as well as the broader public to get a better grasp.
The ELLIS Unit Jena is involving the Friedrich Schiller University Jena, the Max Planck Institute for Biogeochemistry and the German Aerospace Center and is funded by the Carl Zeiss Foundation and the Michael Stifel Center Jena.
OUR RESEARCH WORK
Artificial intelligence and machine learning (AIML) are playing an increasingly important role for modeling and understanding the diverse and interlinked dynamic processes on Earth. Importantly, not only Earth system science benefits from machine learning, but complex Earth system problems can inspire fundamental research in the AIML domain. As in other scientific domains the major challenges relate to interpretability and the integration of machine learning and domain knowledge.
The goal of the ELLIS Unit Jena is thus to combine fundamental development in machine learning (Figure below, rows) with applied challenges concerning spatio-temporal dynamics in the Earth system (Figure below, columns) for a better understanding of the Earth system and its components. An important aspect here is the integration of knowledge into machine learning methods as appropriate assumptions – this can be qualitative knowledge about causal relationships („causal modeling“) or quantitative knowledge about functional relationships, which can be „cast“ into physical, chemical or biophysiological formulas („hybrid modeling“). Causal inference provides a fruitful ground for ML theory and methods, especially for applications in geosciences.
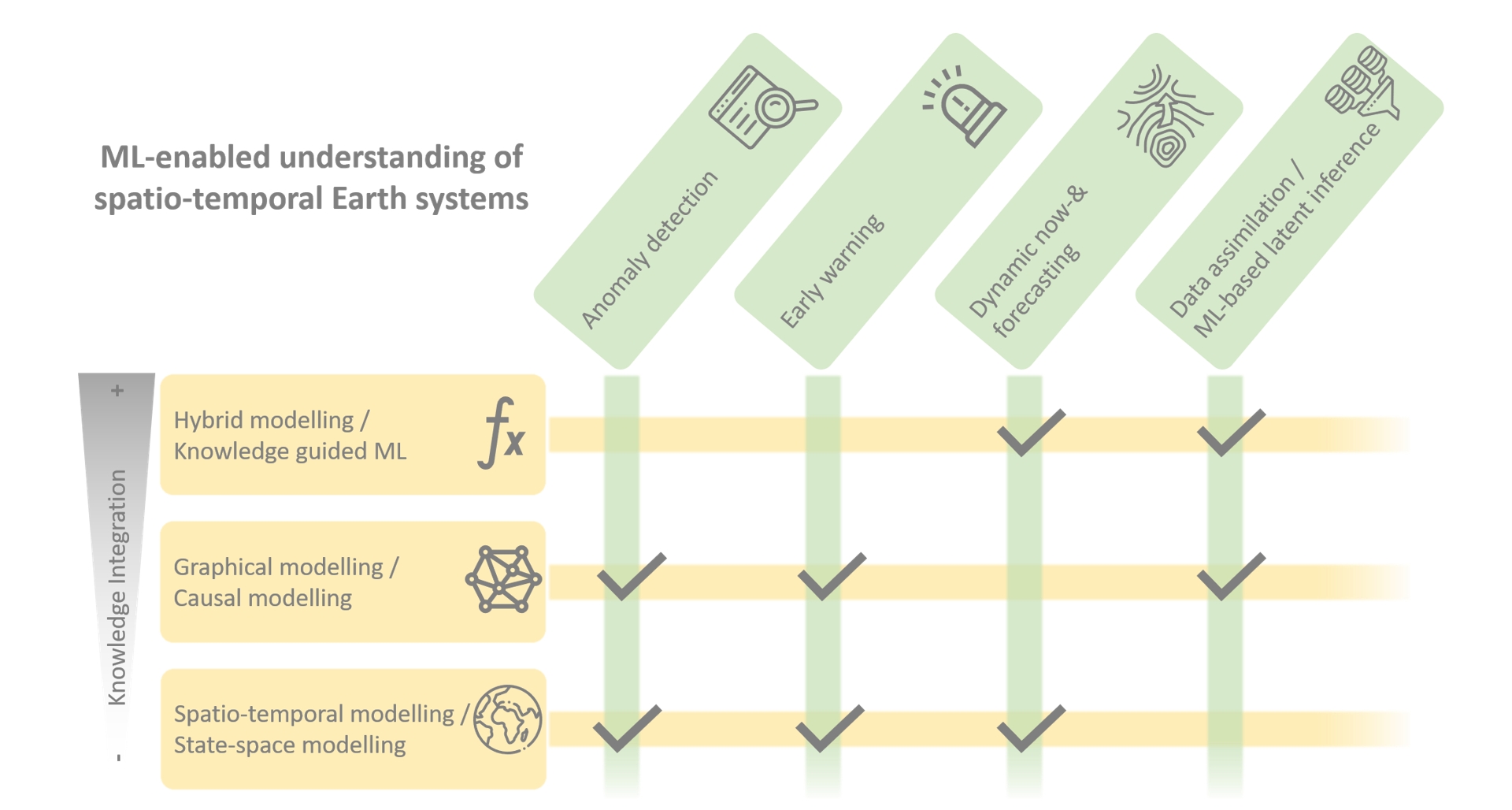
Links between fundamental AIML research and application in the Earth system science. Fruitful bi-directional links in the matrix cells where checkmarks are set.
Causal models are more interpretable by construction and their combination with machine learning constitute a major current research avenue.
A promising approach is to apply causal modeling as basis for explanations, for example, to identify features used for the decision or counterfactuals based on knockoffs. Complementarily, hybrid modeling aims at combining mechanistic models with machine-learning approaches, allowing for physically more consistent predictions and – scientifically more importantly – non-parametric inference of latent variables.
Overall, we identify at least five major challenges and avenues for the successful development and application of machine learning approaches in the geosciences:
Interpretability
Improving predictive accuracy is important but insufficient. Certainly, interpretability and understanding are crucial in this arena, including visualization of the results for analysis by humans. Interpretability has been identified as a potential weakness of deep neural networks, and achieving it is a current focus in deep learning. The field is still far from achieving self-explanatory models, and from causal discovery from observational data. Yet, we should note that, given their complexity, also modern Earth system models are in practice often not easily traceable back to their assumptions, limiting their interpretability as well.
Physical consistency
Machine learning models are adept at fitting observations, but their predictions can sometimes be physically inconsistent or implausible due to factors such as extrapolation or observational biases. By integrating domain knowledge and ensuring physical consistency, we can enhance these models significantly. Teaching the models about the governing physical rules of the Earth system imposes strong theoretical constraints in addition to the observational ones. This approach not only improves the reliability of the predictions but also ensures they adhere more closely to known physical laws, thereby increasing the overall robustness and applicability of the models.
Complex and uncertain data
New machine learning methods are essential to handle complex statistics, multiple outputs, diverse noise sources, and high-dimensional spaces. There is a pressing need for innovative network topologies that can leverage local neighborhoods at various scales and long-range relationships, such as teleconnections. However, the exact cause-effect topologies remain unclear a priori. Incorporating Bayesian and probabilistic approaches will aid in modeling uncertainties, providing a more comprehensive understanding of the data. These advancements are crucial for improving the performance and reliability of machine learning models in tackling intricate real-world problems.
Limited labels
Unsupervised density modeling and semi-supervised learning are promising approaches for Earth system modeling, as they effectively combine the limited number of labeled samples with the vast amount of unlabeled observations. These methods can enhance the accuracy and robustness of models by leveraging the abundant unlabeled data to uncover underlying patterns and relationships. By integrating these advanced techniques, we can improve our understanding and prediction of complex Earth system processes, ultimately leading to more reliable and comprehensive models that better reflect the intricacies of the natural world.
Computational demand
There is a significant technical challenge associated with the high computational cost of addressing current geoscience problems. These problems often involve processing vast amounts of data and running complex simulations that demand substantial computational resources. The scale and complexity of geoscientific data, combined with the need for high-resolution modeling and long-term simulations, exacerbate this challenge. Developing more efficient algorithms, optimizing computational methods, and leveraging advanced technologies such as high-performance computing and cloud-based solutions are essential to overcoming these obstacles.
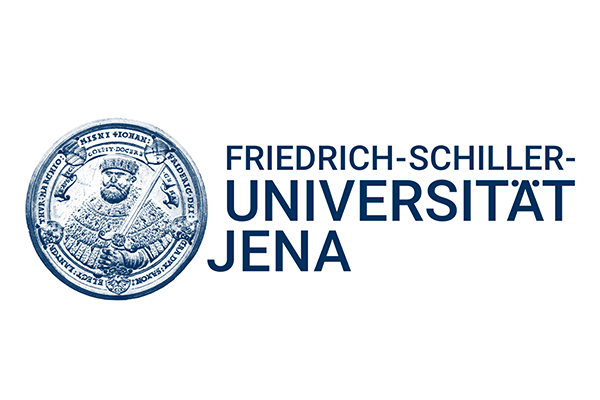
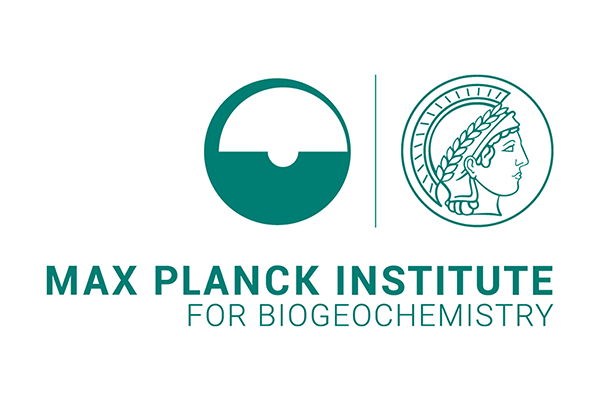
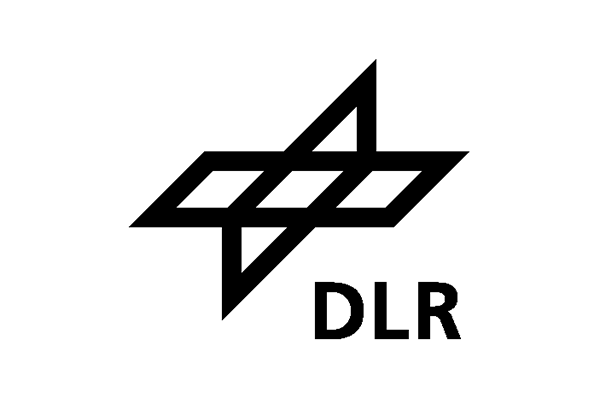
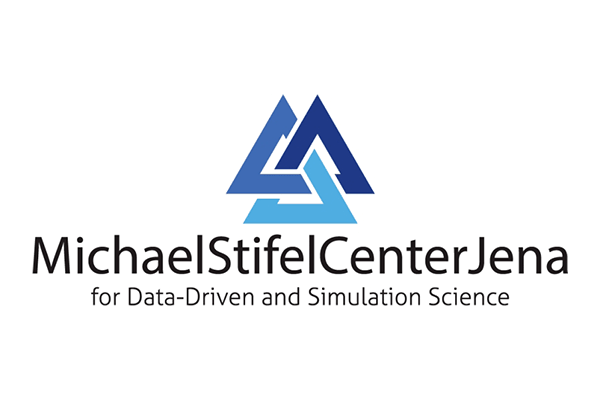
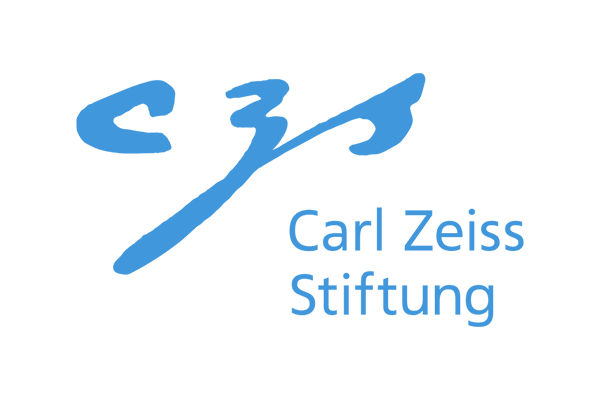
Dr. Conrad H. PHILIPP
European Laboratory for Learning and Intelligent Systems (ELLIS)
ELLIS Unit Jena | Project Coordinator