CZS supported AI projects – session 2
13:20 AM – 14:15 PM
Integrating Knowledge and Machine Learning in Earth System Science
Machine learning models are now making waves in Earth system science, which has traditionally relied on process-based models rooted in first principles. These data-driven models show great advantages in processing large volumes of observational data and modeling Earth system dynamics, with generally superior predictive performance compared to conventional process-based models. However, machine learning models often face several challenges when applied to address specific scientific questions due to their large data requirements, susceptibility to spurious correlations, and difficulty in generalizing to new scenarios. In today’s world, the Earth is undergoing rapid change driven by numerous nonlinear and interconnected processes that remain incompletely understood. Recognizing that reliance on machine learning alone or existing scientific knowledge alone is insufficient for these complex applications, the research community is embarking on an exploration of the synergistic continuum between process-based and data-driven models. Our work at the ELLIS Unit Jena in the CZS project “Knowledge Integration for Spatio-Temporal Environmental Modeling”, is at the forefront of exploring this integrated data-AI-knowledge framework for modeling and understanding the Earth system. In this presentation, we will illustrate the potential of this integration and provide insights into the direction of our research. As we face increasing environmental pressures, we argue that knowledge-integrated AI is not only beneficial but necessary to better understand the complexity of Earth’s interconnected systems.
Speaker and group leader
Shijie Jiang is a group leader at the Max Planck Institute for Biogeochemistry, where he leads an ELLIS Unit Jena research group since 2023. His general research interests include the water cycle, hydrological extreme events, and their interactions with terrestrial ecosystems and climate systems. He specializes in developing knowledge-integrated machine intelligence systems that effectively incorporate diverse scientific knowledge and extensive Earth data into deep learning models for causal understanding. His current research explores (1) Methodologies of scientific machine learning, explainable AI, physics-AI hybrid systems, and causal inference; (2) Coupling and feedback mechanisms between the hydrological cycle and Earth system components; (3) Predictability, attribution, and impacts of extreme hydrological events; (4) Intelligent methods (such as data crowdsourcing, opportunistic sensing) for enhancing Earth system data.
Shijie received his Ph.D. from the National University of Singapore in 2021, where his research introduced computer vision, hybrid models, and explainable machine learning to hydrological monitoring, modeling, and understanding. As a postdoctoral researcher at the Helmholtz Centre for Environmental Research, he conducted investigations on climate features related to compound flood events. He has a solid publication record in Water Resources Research, Hydrology and Earth System Sciences, Geophysical Research Letters, and Journal of Hydrology. Several of his papers have been recognized as top downloaded articles by journals. Shijie also serves as a reviewer for these journals and is the convener of EGU sessions since 2022.
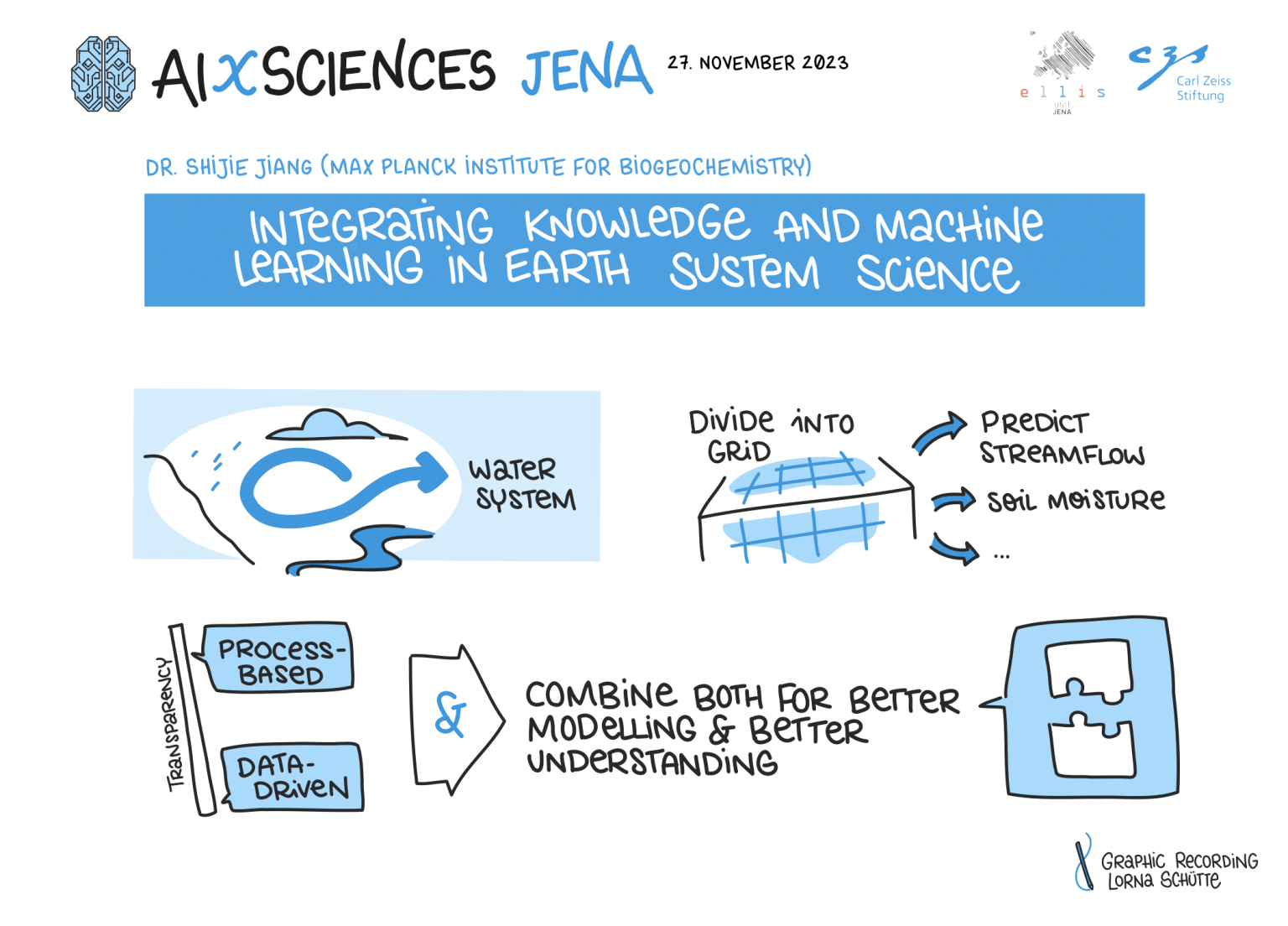
What role should machine learning play in traditional Earth system studies such as hydrology? And conversely, what direction should traditional hydrology take in the era of machine learning? This intersection of machine learning and hydrological studies is the focus of the “Machine Learning for Hydrological and Earth Systems” group. This intersection is not merely about adding machine learning techniques to hydrological studies. Instead, it’s a mutual enrichment: machine learning serves as a bridge, providing deeper insights into the Earth’s nonlinear dynamics through extensive observations, while hydrology contributes a rich repository of domain-specific knowledge to ground machine learning in real-world contexts to ensure it is effective, accurate, and relevant. By combining these two paradigms, we aim to develop the models that not only capture the essence of hydrological and Earth systems, but also emphasize generalization, adaptability across contexts, and causally informed learning. Overall, the group aims to leverage the strengths of both machine learning and domain knowledge to gain a clearer picture of how water interacts with climate, ecosystem, and society.
Principal Investigator
Markus Reichstein is director at the Max-Planck-Institute for Biogeochemistry since 2012 and Professor for Global Geoecology at the Friedrich-Schiller-University Jena. He is also director at Michael-Stifel-Center Jena for Data-driven and Simulation Science and in the speaker board of iDIV representing the Max-Planck-Institutes therein.
His main research interests are related to the interactions between ecosystems and climate within an Earth system context. He addresses this research by combining data-driven machine learning, system modeling and model-data integration approaches.
Markus studied Landscape Ecology University of Münster, received his PhD from the University of Bayreuth in 2001, and carried out research in Viterbo, Missoula and Berkeley with a Marie Curie Fellowship and as Independent Research Group Leader at the Max-Planck-Institute for Biogeochemistry in Jena, Germany.
Markus received several awards such as one of the first ERC grants (Project QUASOM), the Jim-Gray-Seed award for e-science, the Max-Planck-Research Award 2012, and the Piers J. Sellers Mid-Career Award by the American Geophysical Union in 2018 and in 2020 the German Leibniz award as well as an ERCSynergy grant, both related to integrating machine learning with Earth system science. Markus serves as advisory board member for the Helmholtz AI Cooperation Unit (HAICU) and the Tübingen Cluster of Excellence “Machine Learning: New Perspectives for Science”. He is among the few individuals worldwide who is recognized as ISI highly cited researcher in three areas (Environmental Sciences, Geosciences, Agricultural Sciences). According to google his work (more than 140 publications) is cited over 50k times, with an h-index of 103.